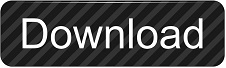
TestRegsubsets = function ( dataset, 圜olName, methods, metrics ) testRegsubsets ( dataset = cleanWhiteDat, 圜olName = "quality", methods = c ( "exhaustive", "backward", "forward" ), metrics = c ( "rsq", "rss", "adjr2", "cp", "bic" )) # Multiple R-squared: 0.3947, Adjusted R-squared: 0.389 # Residual standard error: 0.5752 on 1167 degrees of freedom # Residual standard error: 0.7241 on 3987 degrees of freedom , cleanWhiteDat ) redFit = lm ( quality ~. All of the predictors are numeric values, outcomes are integer.Īs with the whithe wine dataset, the summary stats shows that most of the variables has wide range compared to the IQR, which may indicate spread in the data and the presence of outliers. The variables are the same as for the white wine data set. The red wine dataset has 1599 observations, 11 predictors and 1 outcome (quality). # Get some metrics about the variables summary ( redDat ) # fixed.acidity volatile.acidity citric.acid residual.sugar We remove all the ouliers in our list from the dataset and create a new set of histograms:
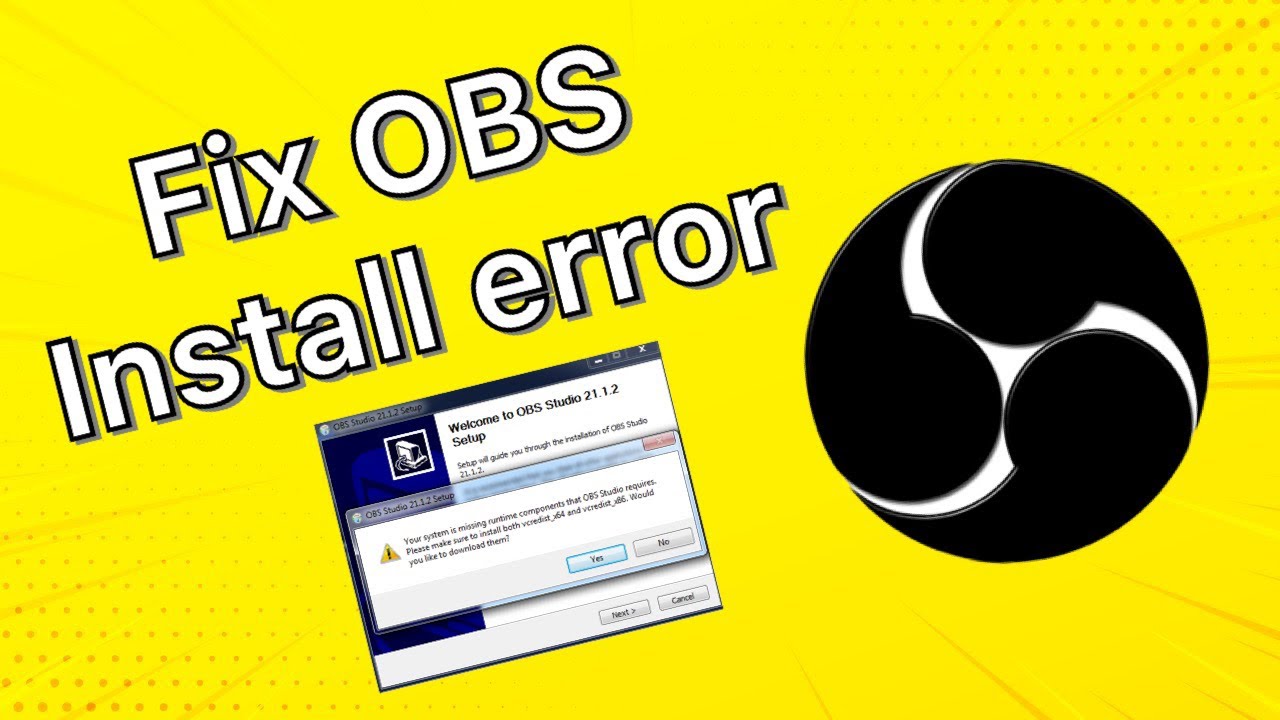
Row 99 and 252 have very high residual.sugar.# density pH sulphatesīy looking at each row we can find out why it is influential: Head ( whiteDat ) # fixed.acidity volatile.acidity citric.acid residual.sugar chlorides We investigate further by producing boxplots for each of the variables: The summary stats shows that most of the variables has wide range compared to the IQR, which may indicate spread in the data and the presence of outliers. All of the predictors are numeric values, outcomes are integer. The white wine dataset has 4898 observations, 11 predictors and 1 outcome (quality).

# Get some metrics about the variables summary ( whiteDat ) # fixed.acidity volatile.acidity citric.acid residual.sugar
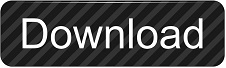